In ancient Greek legend, the Gordian knot was a knot of extreme complexity that tied an ox-cart to a post. Whoever could untie the knot was destined to become the ruler of Asia. When Alexander (later Alexander the Great) was challenged to untie the knot, he came up with an ingenious solution: cut the knot with a sword instead of trying to untangle it.
Health systems are no strangers to complexity, whether in payer agreements, regulatory compliance requirements, vendor contracts, joint venture and other partnership agreements, or physician compensation structures. One area of particular notoriety is funds flow agreements between the teaching hospital, faculty practice plan, and school of medicine within an academic health system. The sheer number and complexity of agreements—some formal, some not—that comprise a typical funds flow model make untangling these agreements a task of true epic proportions.
Artificial intelligence (AI) can provide the sword needed to undo the knot and make transparent the complexities of a funds flow model. Side agreements made over the years may or may not have been officially memorialized, but in most instances they will have left a digital trail, whether in email messages, email attachments, PDF files, Excel spreadsheets, Word documents, or PowerPoints. Mapping this digital trail manually across hundreds or thousands of documents and files would be extremely time-consuming, but an AI language processing model can make quick work of the task, unlocking previously inaccessible financial opportunities.
The key is to identify words that frequently recur in agreements related to funds flow: for example, overhead, benchmarks, medical directorship, corporate services, and academic support. Applying “fuzzy logic,” or what we call semantic similarity, to the language processing model helps ensure that the search also captures related terms. These techniques are also leveraged to determine document similarity, so a collection of related documents can be evaluated quickly based on their content. With the relevant documents identified, it is possible to understand the history of how the funds flow model has evolved, and whether that evolution was based on a legal agreement, a side agreement, or a handshake. It also gives clarity to what is happening on the ground versus what has been legally agreed to in the funds flow model. The gap can be significant, amounting to tens of millions of dollars.
Most significantly, this information lays the groundwork for restoring the funds flow model to what has been legally agreed to or renegotiating and formalizing a new funds flow agreement. By understanding the nature of different fund flows (e.g., a legal agreement versus a side arrangement) and the amounts of those fund flows, the teaching hospital (which is the primary source of funds in almost all funds flow models) can identify where the greatest opportunities and the strongest negotiating positions exist (See Figure 1).
Figure 1: A Framework for Prioritizing Funds Flow Opportunities
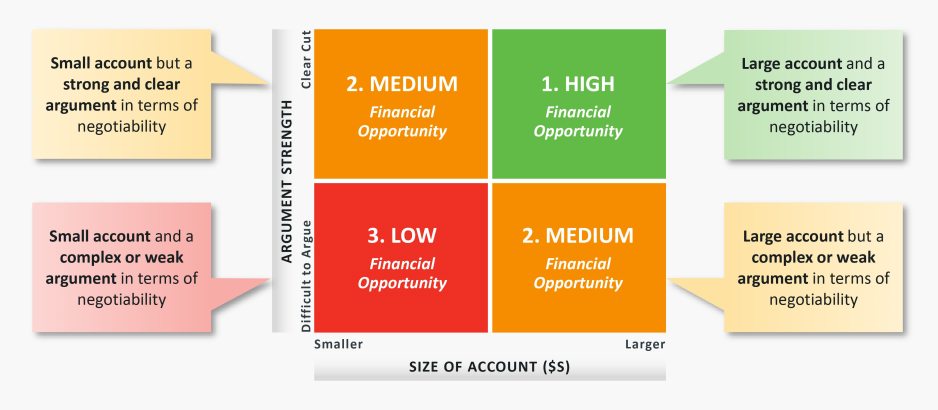
In this case, we applied AI in the form of natural language processing to an academic funds flow model, but a similar approach could be used for any agreement that has evolved, officially or unofficially, over the years. Within our funds flow example, some of the greatest opportunities were within areas of provider compensation: payment for more FTEs than formally agreed to, payment for more hours than providers worked, payment for providers who were missing activity within the electronic health record, and double-payment for advanced practice provider (APP) work where, for example, the rendering provider was an APP but the billing provider was a physician or a different APP. With many health systems facing a growing problem with subsidization of their physician practices, understanding potential gaps between agreed upon compensation structures and “on the ground” realities could generate real savings.
AI itself can seem like a technology of bewildering complexity, which can make it difficult to understand the types of problems it can solve. In our experience, it can provide a pragmatic and efficient tool for cutting through confusion and bringing clarity to arrangements where significant funds may be at stake.